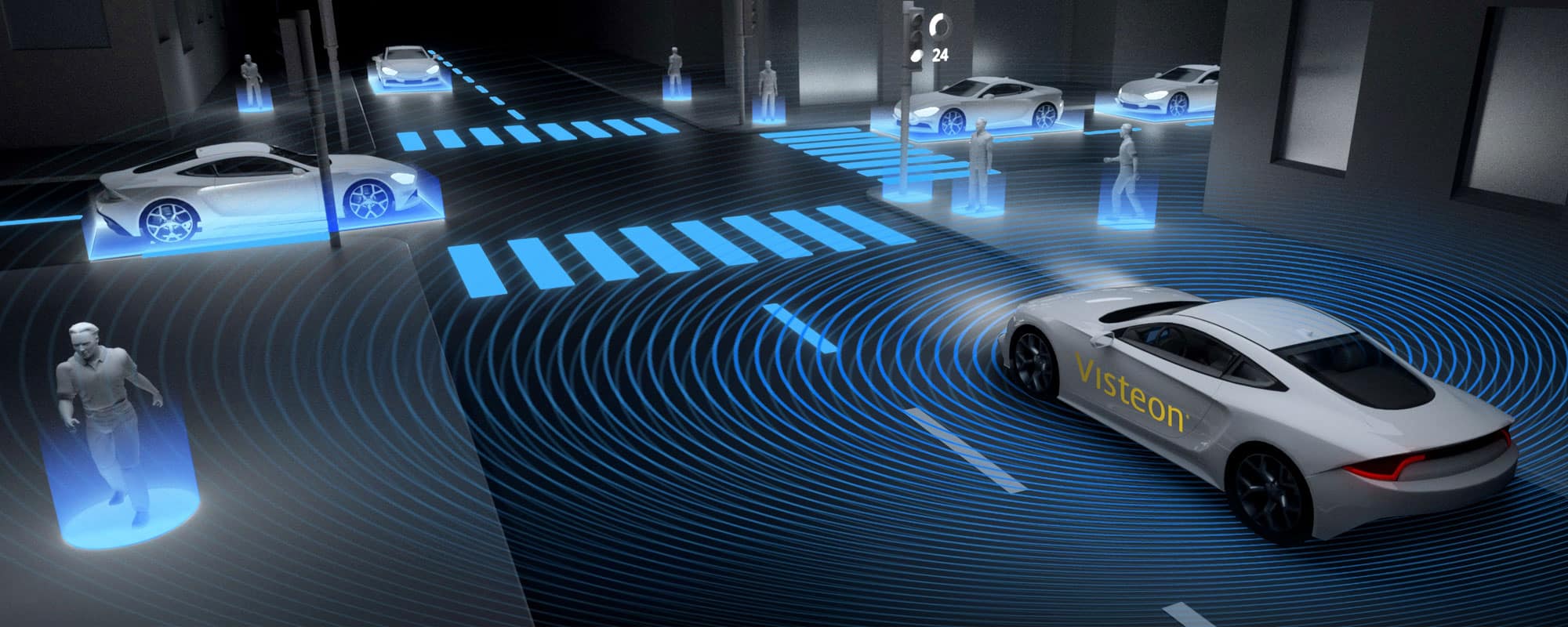
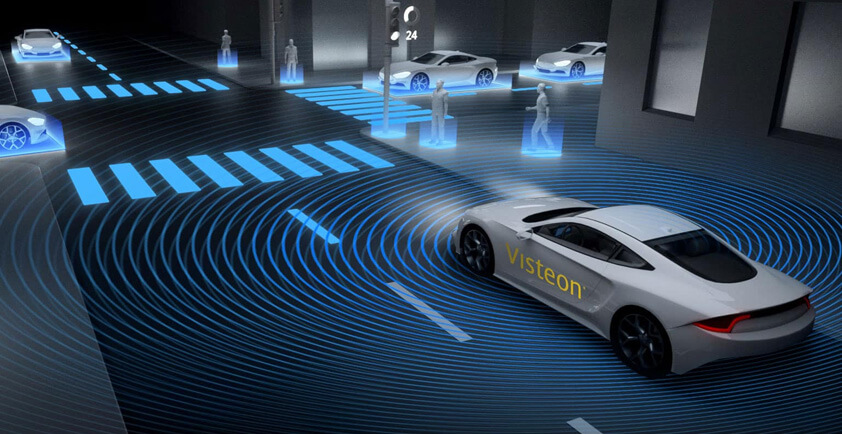
VISTEON ADAS EXPERTS PRESENT REPORT ON AUTONOMOUS DRIVING DEVELOPMENTS
For the past decade, assisted and autonomous driving have been envisioned as the future of mobility – with the ultimate ambition of its development to eradicate human error in order to mitigate the number of road accidents. To achieve this objective, the auto industry has pursued investigations and demonstrations on test vehicles but maturity in terms of safety has been undermined due to several factors. These include the level of precision in the information perceived by deployed sensors and the lack of computation power to execute sophisticated camera-based detection algorithms - while at the same time applying sensor fusion techniques on the information from various sensors.
In a new report, a team of ADAS experts from the Chief Technology Office (CTO) explains the main frameworks directing autonomous development and charts the evolution to date in the paper entitled: “Autonomous Driving – A Bird’s Eye View”.
In recent years, there has been tremendous progress in the area of autonomous driving. One explanation is that the auto industry has pioneered the art of acquiring precise information by fusing data available from different sensors - namely camera, radar, LiDAR, ultrasonic, inertial measuring unit (IMU) and global positioning system (GPS). The fused information is encapsulated in the form of an environmental model recognized by the vehicle, which includes the information concerning free space, lanes, traffic regulation and moving objects. Essentially, the environmental model serves as a platform that allows us to realize different functions for assisted driving (ADAS).
Taking these factors and past limitations into account, Visteon’s DriveCore™ Compute hardware solution promises both sufficient bandwidth and the computation power to process information from the multiple sensors that are required to achieve scalable levels of autonomous driving.
"Autonomous driving demonstrates a typical scenario of human-machine interaction (HMI) where the driving tasks are shared between the driver and the system. Its development can be misleading unless a common terminology is established within the industry - thus, leading to the precise definition of the boundary in terms of responsibility shared between the human and the system. The Society of Automation Engineers (SAE) has aimed at solving this problem by classifying autonomous driving into different levels, from 0-5." Vikram Narayan, Head of Computer Vision
It is clear that the vehicle is equipped with different sensors that contribute to the environmental model. To illustrate this aspect from an ADAS point-of-view, an automated lane change (ALC) is presented as an example. To execute an ALC, the system needs to ensure that there are no moving objects in the blind spot. Not only that, the ALC must evaluate the time to collision for each moving object that may appear in the blind spot while the target vehicle is transitioning to the adjacent lane.
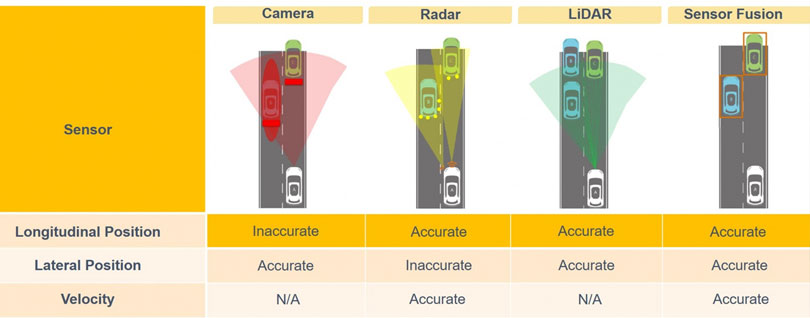
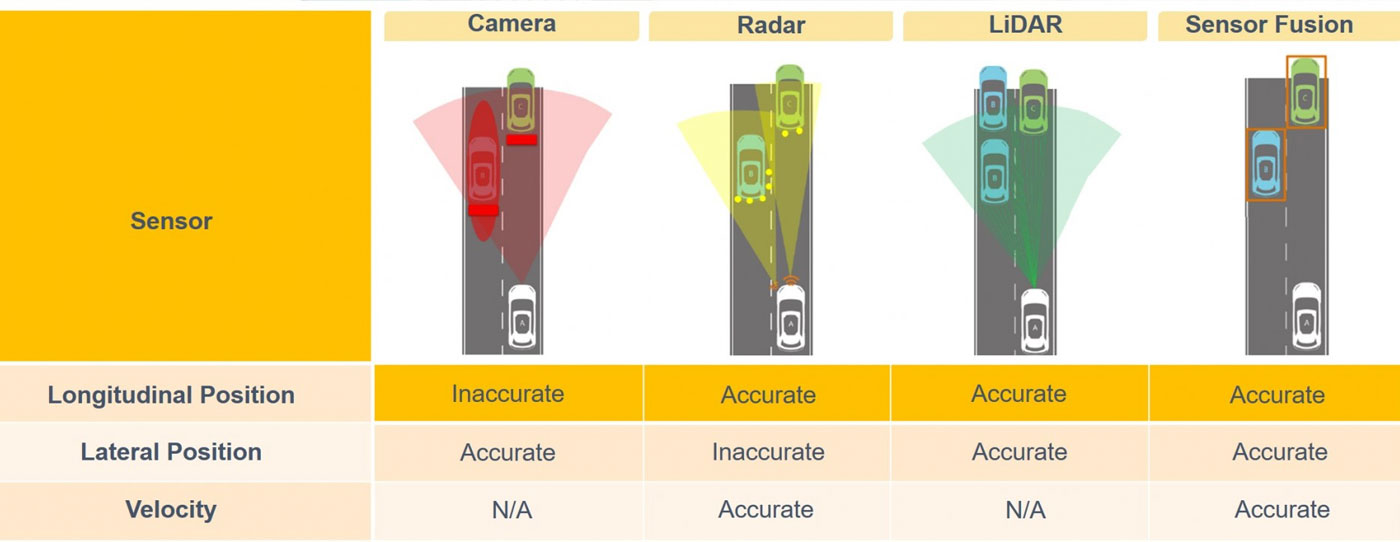
The ALC then utilizes the environmental model to procure information for moving objects specifically (lateral and longitudinal) position and velocity relative to the target vehicle - since individual sensors either cannot detect position and velocity or the detection itself is not sufficiently accurate. This is where sensor fusion comes into the picture, allowing us to improve the accuracy of the perceived information.
Every ADAS function consists of numerous situations that are critical from a safety standpoint. One situation concerns the lane change initiated by the driver of the ego-vehicle (vehicle A) which is most likely to happen when a slow vehicle (vehicle C) is encountered in the ego-lane. However, whenever a lane change is implemented the system must cater for an approaching vehicle (vehicle B) that may appear in the adjacent lane.
Next, it becomes essential to quantify the distance so that the situation is handled appropriately. This can be achieved, for instance, by establishing the closing speed that accounts for the relative speeds between vehicles A and B allowing a comfortable braking distance for vehicle B – while at the same time maintaining a safe distance from vehicle A as prescribed by traffic regulations. The closing speed can be used as a parameter to depict the sensor requirement.
Despite strong presence in the market for several years, autonomous driving – with reference to higher SAE levels – is still an unexplored asset for a large portion of the automotive industry. That said, the market trend clearly states that ADAS is currently, and shall continue for the upcoming future, the force driving the automotive industry.