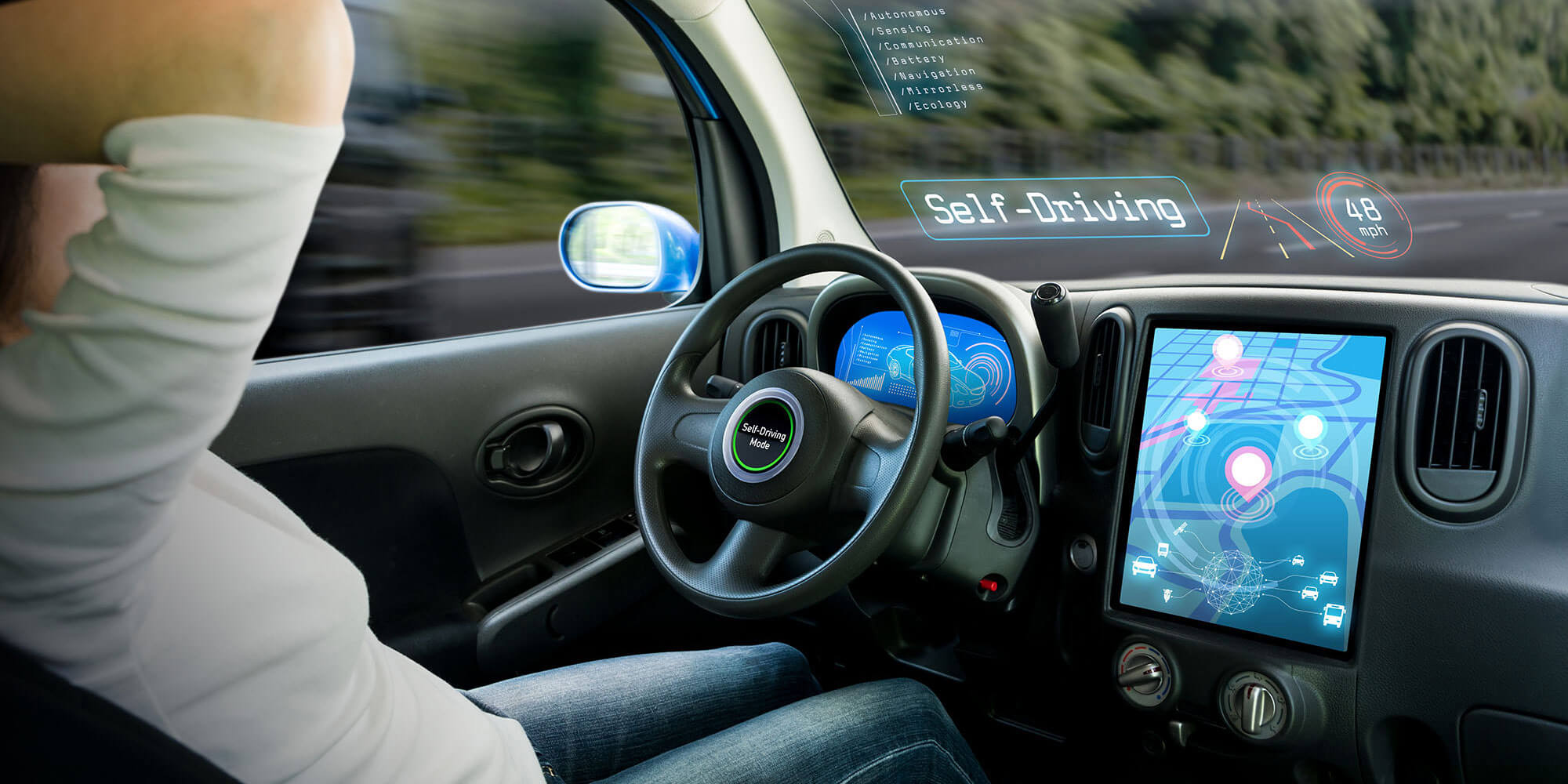
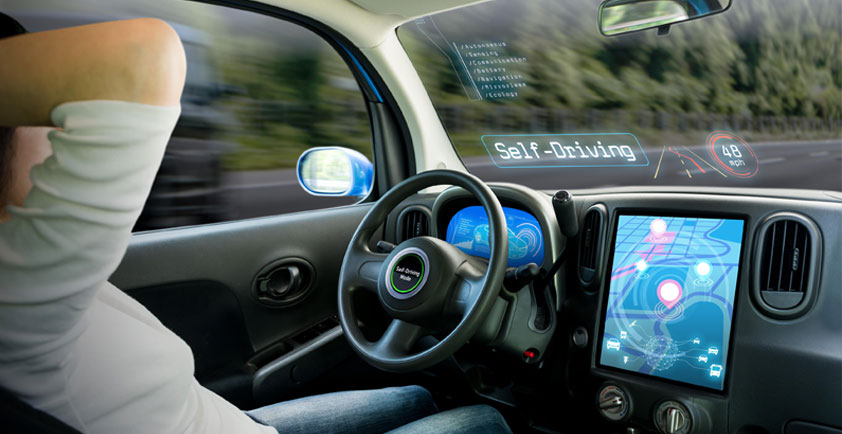
U-BLOX: AUTONOMOUS CARS AND OVERCOMING THE INADEQUACIES OF ROAD MAPPING
Functional safety standards for self‑driving cars require redundancy in case any of the sensors - LiDAR, radar, cameras, etc. - fails. While sensors such as radar and LiDAR may provide redundancy for object detection, when it comes to cameras only a highly accurate map can serve as the source of redundancy, helping the autonomous vehicle make the right decisions by comparing their visual glimpses of the world with a map stored in memory.
What’s required is not just any map, these cars need a three‑dimensional representation of the environment around them, one that is updated continuously and is accurate down to the decimeter. These detailed maps, sometimes called "HD" maps, will contain things like images of the road surface and surroundings, including the location of trees, hydrants or other physical objects in the environment.
But the inadequacy of road mapping remains among the problems still to be overcome. Such maps are being developed, but collecting accurate 3D data of cities and keeping the information on them up‑to‑date have both historically been incredibly expensive and time‑consuming; mapmakers are walking the tightrope of cost versus benefits to achieve the necessary quality at a reasonable price. What is more, HD maps are only useful if the data underpinning them is fresh. So it is necessary to minimize the gap between the moment a road change happens and the time it is reflected in the map.
It seems clear that the maintenance of such maps will not be possible at the required update rate without some sort of crowdsourcing approach. Crowdsourced data can come from cameras or radar sensors on customer vehicles, as well as from third parties that want their drivers to obtain the features needed in local maps - a set of landmarks put together attached to the roads - in order to take advantage of the connectivity of the road network infrastructure. Trouble is, as it cruises through the streets, a self‑driving car can collect more than a terabyte of data a day to be sent to the cloud. With that much detailed information coming from the car’s many sensors it is unrealistic and uneconomical to try to send it through a network like the Internet.
HD maps include both things that don’t change very often such as notations about lane width, placement of stop signs, signals and crosswalks as well as elements that need updates to reflect current road status. Not having information about the slightest variation on the road, such as a new construction project that starts overnight and any temporary rules that accompany them, could have calamitous implications.
These maps differ from Google Maps, Apple Maps, etc. in that they are intended for machines rather than humans. Maps for humans can afford to be a little imprecise because, even though the map doesn't know the difference between a sidewalk and a street, you do.
Eventually, all self‑driving vehicles will contribute to a collective database that updates in near real‑time. Mobileye has already promised to create such a database with its Road Experience Management (REM) platform camera data coming from its partners including Nissan, Volkswagen and BMW. Mobileye will be gathering data from three million vehicles’ EyeQ4 forward‑facing cameras, which will be compiled into a database that will be usable for driver assist and self‑driving systems.
When a car knows precisely where it is, it can follow predetermined routes in its memory, simplifying the driving process. As such, Global Navigation Satellite System (GNSS) based absolute positioning will remain at the core of autonomous driving. High Precision GNSS technology is capable of providing self‑driving vehicles with decimeter‑level accuracy to ensure a vehicle stays in its lane, or a safe distance from other vehicles.
The need for HD maps means that no matter how advanced a company’s self‑driving technology, it must partner with someone capable of making a detailed map of every road on which self‑driving vehicles will drive. This effort is going to require new types of collaboration and new partnerships between companies that may be at disparate ends of the autonomous vehicle ecosystem.
For example, Momenta and Analog Devices (ADI) have a strategic collaboration to advance the development of high‑definition (HD) maps in autonomous vehicles. As part of the collaboration, Analog Devices’ inertial measurement units (IMU) will be used to improve the mapping system setup and technology upgrades in Momenta’s L3 freeway ramp‑to‑ramp solution and L4 full autonomy driving solutions. Under poor weather conditions, or when a GPS signal is lost, an IMU becomes essential in delivering high precision mapping output.
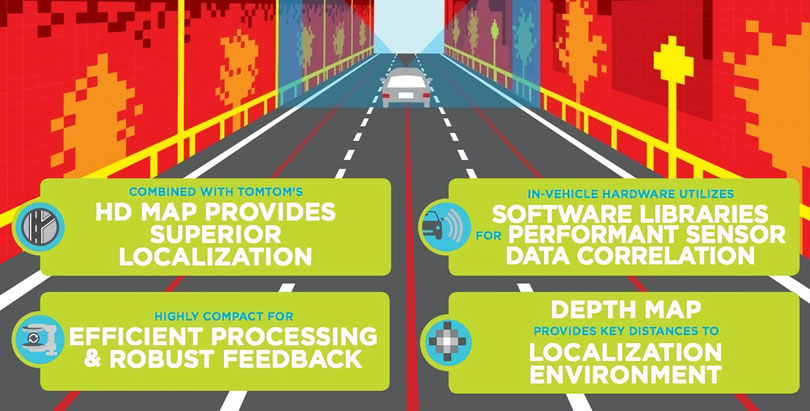
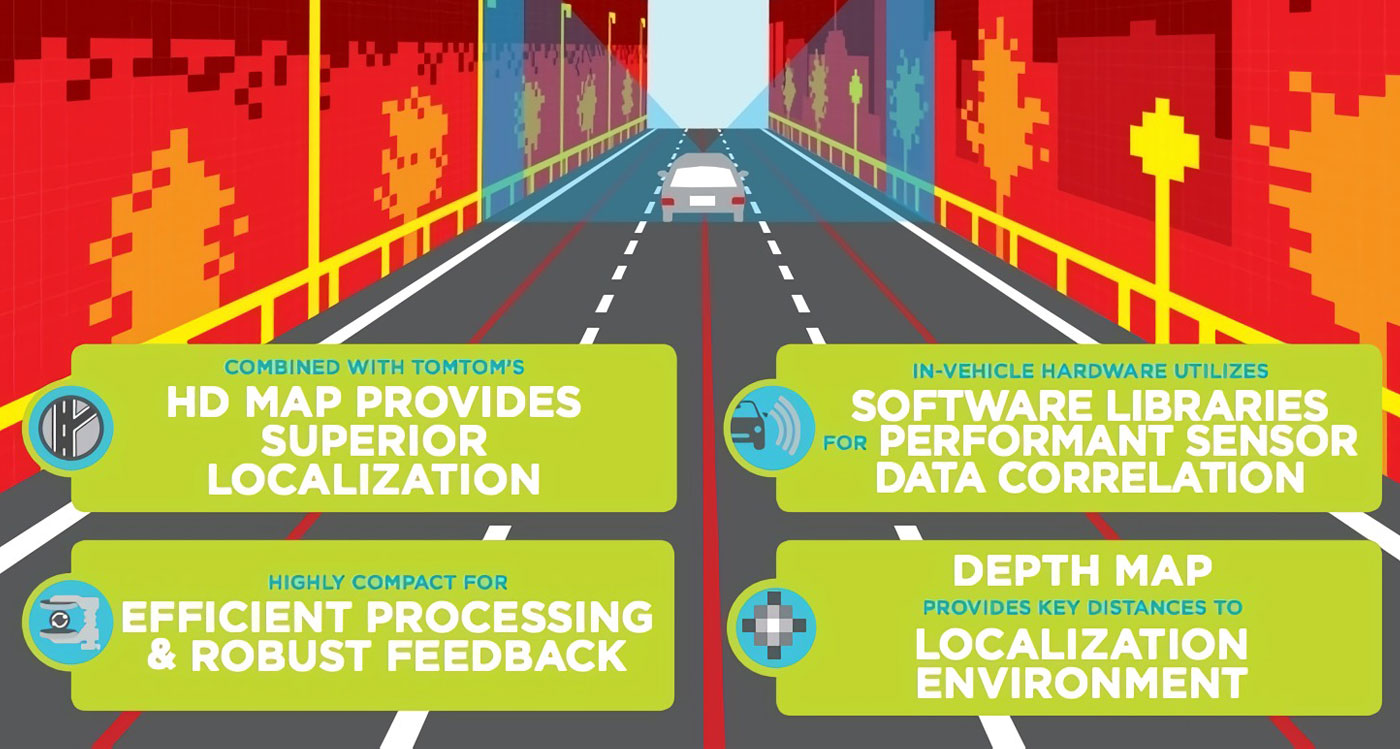
Location technology specialist TomTom is collaborating with Denso on its software platform for autonomous vehicles. TomTom’s HD map will work in combination with Denso in‑vehicle sensors such as cameras and radars to power the localization, perception and path planning functions for a complete autonomous driving system. As part of this agreement, Denso will provide processed sensor data to TomTom’s end‑to‑end mapping system for autonomous vehicles and Advanced Driver Assistance Systems (ADAS), updating TomTom’s HD map on‑the‑fly. This will be achieved by generating crowdsourced map updates called Roadagrams which are used to ensure the HD map reflects the reality of the road.
To obtain detailed maps suitable for autonomous driving LiDAR manufacturer Velodyne recently acquired mapping and localization software as well as intellectual property assets from Mapper.ai. Mapper technology will enable Velodyne to accelerate development of Vella software for its directional view Velarray LiDAR sensor. Velarray is the first solid‑state Velodyne LiDAR sensor that is embeddable and fits behind a windshield.
In Japan, Dynamic Map Platform Co. has acquired all shares of US‑based Ushr, Inc. and will begin to provide high definition 3D digital maps with 15 cm absolute accuracy and <1 cm relative accuracy to a wide range of users, not only in the Japanese market but also in the North American market. General Motors has integrated Ushr’s HD Map into its Super Cruise system, which itself was a first‑to‑market feature for passenger vehicles. Ushr’s current database covers about 200,000 miles of controlled‑access highways in North America; the amount of roadway that changes in that database is said to be between 6,000 and 8,000 miles a year.
All systems providing actual road conditions in near‑real time for use by a self‑driving vehicle or ADAS software depend on knowing where the car is at all times. Advanced GNSS devices can help in providing high‑definition 3D street maps and deliver large‑scale mapping of geographic terrain, offering location coordinates with sufficient accuracy to enable all of these essential applications.
Learn more about GNSS solutions at u‑blox.
Author: Murray Slovick - Editorial Director and Principal of Intelligent TechContent