
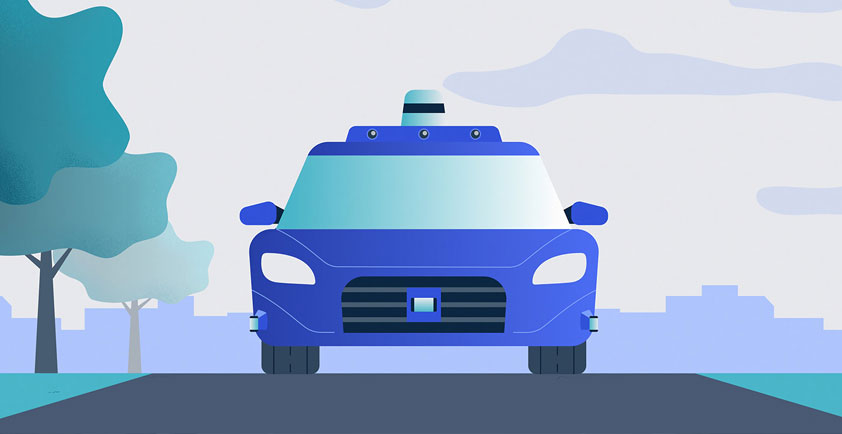
TEACHING CARS TO SEE WITH AI
The future looks bright for smart and safe transportation as the core technologies required for autonomous driving are developing rapidly. But what does it take for a car to see and safely navigate the world? At Qualcomm Technologies, we’ve been actively researching and developing solutions that lay the foundation for autonomous driving.
Diverse sensors complement each other for autonomous driving
Just as humans perceive the world through their eyes, ears, and nose while driving, cars are learning to perceive the world through their own set of diverse sensors. These sensors, such as the camera, radar, lidar, ultrasonic, or cellular vehicle-to-everything (C-V2X), complement each other since each sensor has its own strengths.
Cameras are affordable and can help understand the car's environment, like reading text on a road sign. Lidar creates a high-resolution 3D representation of the surroundings and works well in all lighting conditions. Radar is affordable and responsive, has a long range, measures velocity directly, and isn’t compromised by lighting or weather conditions. A car can see best when utilizing all of these sensors together, otherwise known as sensor fusion, which is further discussed below.
Machine learning research to make radar more perceptive
Each of these sensors is becoming increasingly cognitive, allowing vehicles to better understand the world, so that they can navigate autonomously. One sensor that is expected to be invaluable for self-driving vehicles is radar. We wondered if we could make radar even more useful with AI.
With radar, the receiver captures reflected radio waves. Traditional radar algorithms reduce the received signal to a sparse point cloud, and then analyze it to draw conclusions about surrounding objects. The problem with this process is that a lot of details get lost in the data reduction. So, our AI research team set out to find a way to analyze the raw radar signal directly.
We found our solution in machine learning. By applying machine learning directly to the radar signal, we’ve improved virtually all existing radar capabilities, enhanced overall vehicle “sight,” and taught radar to detect both objects and their size. For example, we expect AI radar to be able to draw a bounding box around difficult-to-detect classes, such as bicyclists and pedestrians.

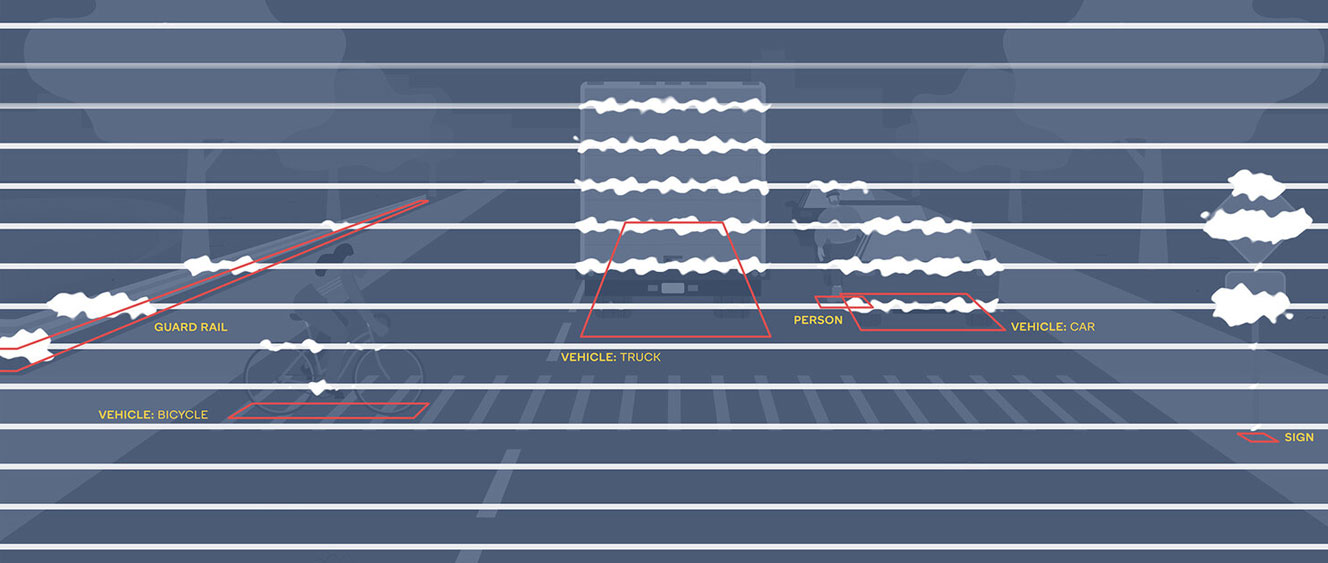
From this breakthrough achievement, AI and radar can help make a difference in split-second decisions on the road. Check out the video and read our paper “Vehicle Detection With Automotive Radar Using Deep Learning on Range-Azimuth-Doppler Tensors” to learn more.
Sensor fusion harnesses the value of diverse sensors
Beyond radar research, we’ve also explored sensor fusion for autonomous driving. Sensor fusion is not new for us. We’ve done sensor fusion for drone navigation, XR head pose, and automobile precise positioning. For autonomous driving, sensor fusion is about getting a precise and real-time understanding of the world to make critical decisions.
This can be very challenging in an automotive environment where there are many fast-changing variables, ranging from weather and road conditions to varying driving rules and speed limits. In the paper “Radar and Camera Early Fusion for Vehicle Detection in Advanced Driver Assistance Systems”, we showcase the results of early fusion of camera and radar data using AI. These two sensors are highly complementary – for instance, current radars can give absolute distance estimates, but not height of the object, whereas camera is exceptionally good at telling us object height for a given distance.
Traditional fusion algorithms perform late or object-level fusion; they detect objects in the two sensors separately, and then try to match them across the two sensors and fuse their properties. The drawback of this is that the object features are generally not available in the matching process, leading to poor matching and fusion output. In our approach, we start out with minimal feature extraction on both sensors and fuse the features early on, allowing the AI to use features from both the matching and the final fusion output. Thus, the complementary capabilities of the two sensors are used more effectively, leading to better detection of 3D objects.
Paving the road to autonomous driving
Improved perception and fusion from diverse sensors create a more robust understanding of the environment, leading to better path and motion planning. Our AI and automotive research teams work on all aspects of the ADAS full-stack to support a smoother driving experience.
Our research is not meant to stay in the lab. At Qualcomm AI Research, we quickly commercialize and scale our breakthroughs across devices and industries, reducing the time between research in the lab and offering advances that enrich lives. For example, the recently introduced Qualcomm Snapdragon Ride Platform — which combines hardware, software, open stacks, development kits, tools, and a robust ecosystem to help automakers deliver on consumer demands around improved safety, convenience, and autonomous driving— already includes some of our research. Our AI automotive initiatives will hopefully contribute to saving lives in our intelligent, connected future.
Author - Radhika Gowaikar, Sr. Staff Engineer, Qualcomm Technologies