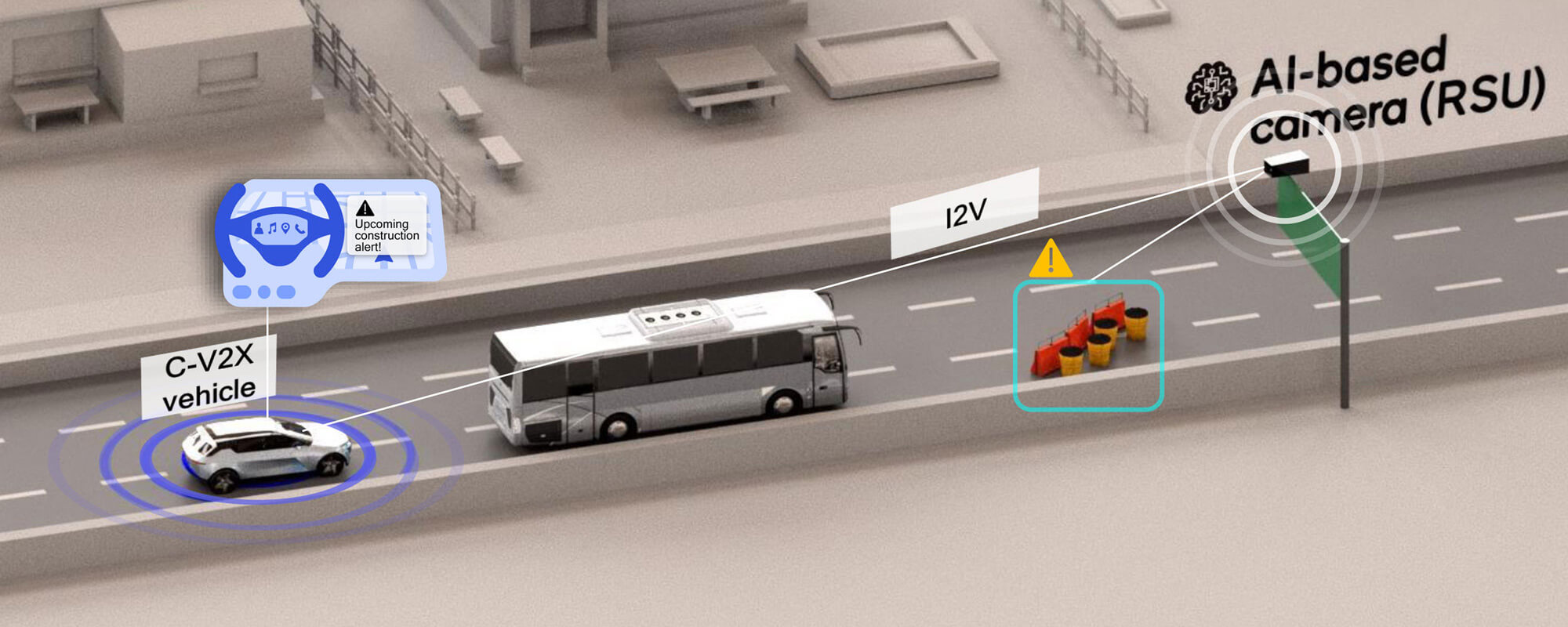
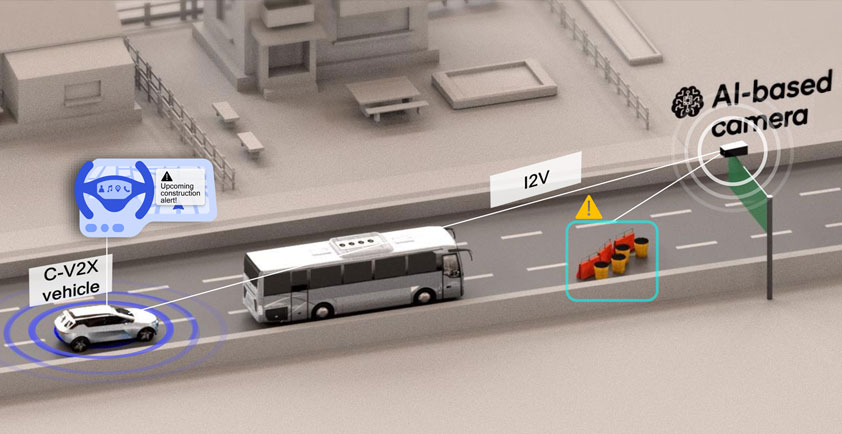
IS VEPP A MORE ACCURATE AND AFFORDABLE AUTOMOBILE POSITION-LOCATION TECHNOLOGY?
Automakers are rapidly taking their vehicles to the next level of connectivity and intelligence. And with breakthroughs such as C-V2X gaining momentum, auto manufacturers will need to source highly reliable and accurate position-location technology to ensure that vehicles safely and reliably interact with connected things such as other vehicles, road infrastructure, mobile devices in the hands of pedestrians and cyclists, and probably more. However, systems such as high-precision GNSS/INS system can cost tens of thousands of dollars, which prevents them from being adopted for broad commercial deployments.
In an effort to transform and accelerate the automobile industry and make roads safer for all, we recently developed Qualcomm Vision Enhanced Precise Positioning (VEPP) technology, which fuses multiple automotive sensors – Global Navigation Satellite Services (GNSS), a camera, an Inertial Measurement Unit (IMU), and wheel sensors — to offer more accurate global positioning of vehicles. Equally important for auto manufacturers is that VEPP is relatively affordable, using sensors already present in today’s vehicles.
VEPP accuracy and possible use cases
Our VEPP testing achieved lane-level accuracy — accurate to <1 meter. In comparison, GNSS-based solutions, possibly incorporating IMU and wheel sensors, can only achieve this type of accuracy in open-sky conditions. Furthermore, the GNSS-based solutions can be challenged in urban environments due to satellite signals encountering multi-path propagation, resulting in errors of several meters — quite critical in driving situations. VEPP, on the other hand, in such scenarios has demonstrated that a single camera — common in today’s new vehicles — is designed to play a critical role in achieving lane-level accuracy in GNSS challenged environments. VEPP does not have any dependency on the availability of any kind of map.
What’s the significance of lane-level accuracy? It enables a host of applications, critical to near-future automobile capabilities:
> Lane-level navigation: in contrast to current navigation systems, lane-specific guidance can be provided. For example, VEPP is designed to help identify that the vehicle is on an exit-only lane, or a turn-only lane, and thereby guide the user to change lanes in order to stay on the road. Likewise, VEPP is designed to help determine the number of lanes that the vehicle needs to change in order to take an exit.
> Lane-specific C-V2X warnings in urban areas: for instance, a broken-down car could alert approaching cars that it has stalled, or traffic signals could “tell” waiting cars time to green in either left-turn or drive through lanes.
> Autonomous driving: requires vehicle location on a high definition (HD) map comprised of lanes and signs. Because of its accuracy, VEPP is designed to greatly simplify the processing step of associating lanes and sign information observed in the vehicle camera with corresponding landmarks in the HD map. This association step is critical for locating the vehicle to the sub decimeter accuracy, demanded by Level 3 and higher autonomy.
> HD map crowdsourcing: as mentioned above, a critical component for autonomous driving is the availability of HD maps. In addition to generating these maps, there is the need for constantly updating maps due to changes in roadways. This is currently possible only through crowdsourcing of vehicle positions and road observations to the cloud for processing. VEPP’s accuracy offers simpler processing in addition to generating maps tethered to world coordinates.
To demonstrate VEPP, Qualcomm Technologies developed a board (see video below, 0:15-0:19) that consists of multiple components: a front-facing camera, an Inertial Measurement Unit (IMU), and a single frequency (L1) GNSS receiver1 connected to a vehicle Controller Area Network (CAN) bus. VEPP uses wheel sensor information from the CAN bus, specifically wheel speeds and steering angle. It also uses a Kalman filtering framework whose implementation is optimized on Qualcomm Snapdragon automotive processors.
VEPP versus competing solutions
Based on our testing, Qualcomm Technologies strongly believes that VEPP is a better solution than many competing technologies, including standalone differential GPS and high-precision GNSS/INS.
VEPP vs. a differential GPS receiver
We first compared the performance of VEPP verses differential GPS2 while driving in an urban area with tall buildings.
In the video (between 0:38 – 0:52), we drove a car using VEPP, running in real time on a Snapdragon processor, to output a global position. This is shown in blue dots, overlaid on Google Maps. Simultaneously, we computed the output position using only differential GPS signals, shown in red dots.
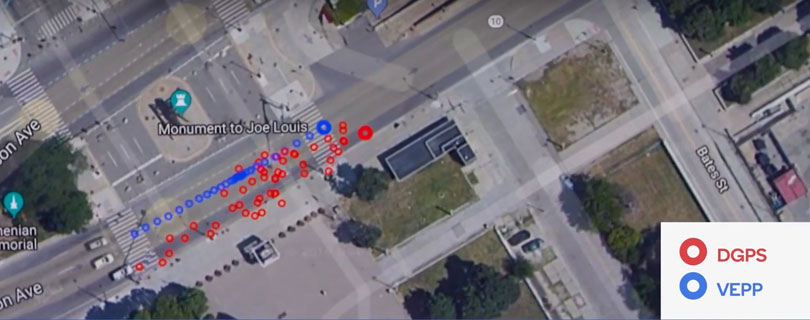
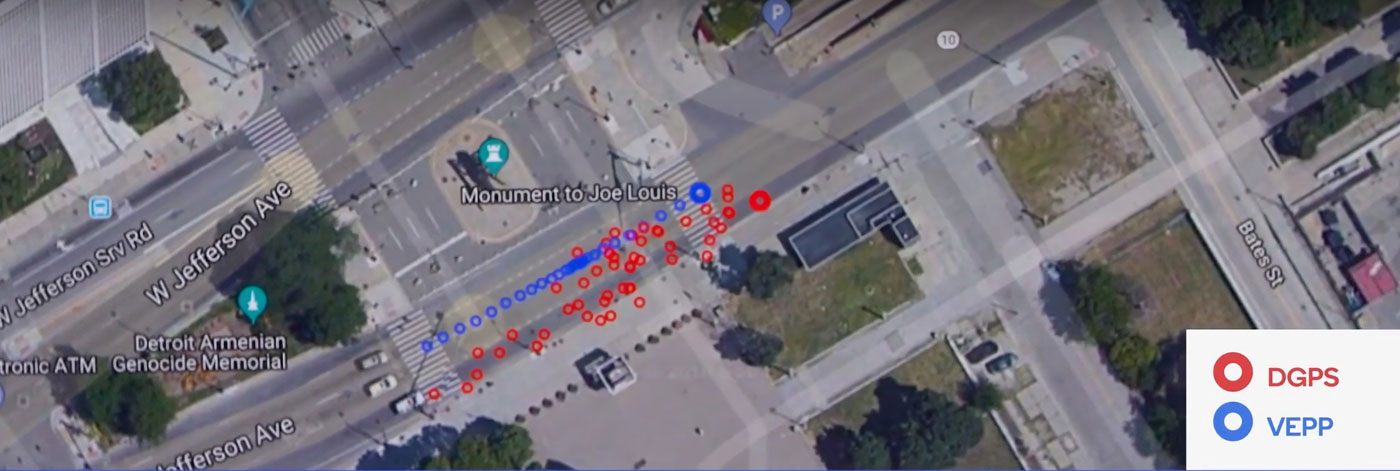
Differential GPS positioning delivered “bouncing” red dots, resulting in errors of several meters, whereas VEPP positioning delivered a single lane of blue dots.
VEPP vs. a differential GPS receiver and a high-precision survey-grade GNSS/INS receiver
Next, we compared the performance of VEPP verses a differential GPS receiver and a survey-grade high-precision GNSS/INS receiver inside a parking garage.
In the video (0:57 – 1:21), VEPP position estimates are shown in blue dots, the differential GPS in red, and the survey-grade GNSS/INS in green. Note that the VEPP estimates stay tightly within the driving area, while the differential GPS’ estimates are inaccurate due to poor reception of GPS signals within the garage, the GNSS/INS’ estimates3 are observed to not stay within the driving area.
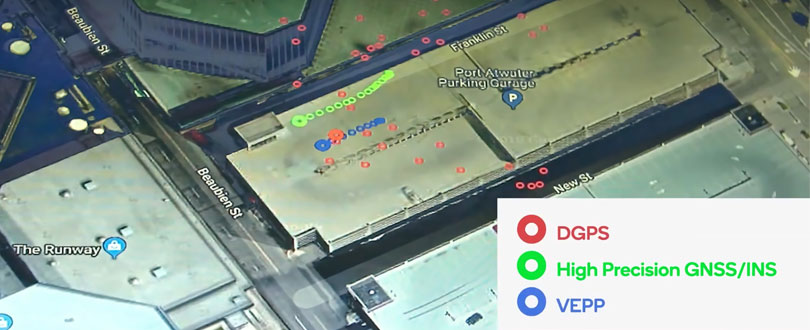
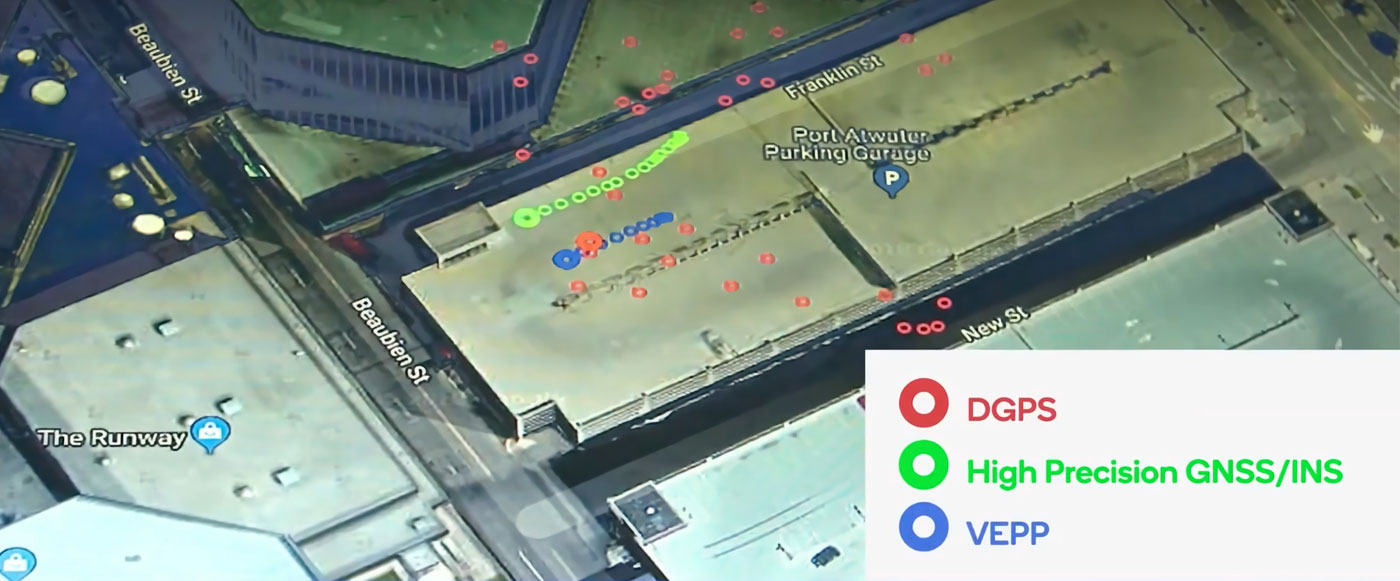
It’s important to note that VEPP’s accumulated error over the entire drive route in the parking garage is on the order of 0.1% of the distance travelled. This represents a significant improvement over dead-reckoning solutions available today.
The key to VEPP’s performance is fusing all sensors at the measurement level, leveraging their complementary nature. In open-sky, the algorithm relies heavily on GNSS, while the camera and wheel sensors are critical in urban areas. An added benefit of using the camera is that it assists in calibrating the IMU in multi-path scenarios. This is the reason for the improved dead-reckoning performance in GNSS-deprived environments, such as a parking garage.
VEPP is a high-precision, low-cost positioning solution for auto manufacturers
In summary, VEPP is an extension of the capabilities that Qualcomm Technologies has brought to the automotive market over the last 15 years, starting with:
> the communication element in support of telematics services,
> the integration of GNSS capability into the telematics platform, reducing the cost of getting GNSS support in the car,
> and now facilitating a host of new applications that require lane-level accuracy in the most challenging environment previously only supported by high-end industrial solutions not suitable for deployment in mass-market cars.
Leveraging our considerable expertise in both GNSS and computer vision, we believe that we are the first in the industry to demonstrate VEPP, and that next-generation navigation applications demanding lane-level positioning, C-V2X platforms seeking support for advanced use-cases in more challenging environments, and all levels of autonomous driving applications stand to benefit from the adoption of Qualcomm VEPP.
In the case of C-V2X deployment, functionality is expected to be implemented in a standalone C-V2X box or in the Telematics Control Unit (TCU) in vehicles. With higher computation capabilities in the TCU, Qualcomm Technologies brings in VEPP as an extension of current cutting-edge Qualcomm Technologies location solutions. This will require facilitating access to the camera sensor for the C-V2X box/TCU, in addition to addressing considerations related to camera placement, such as on or near the roof antenna, for efficient cabling.
Interested customers/parties who have requirements for lane-level position accuracy should reach out to the Qualcomm Technologies Automotive Product Marketing team for more in-depth discussions on target applications and associated requirements.
Anshuman Saxena - Director, Product Management