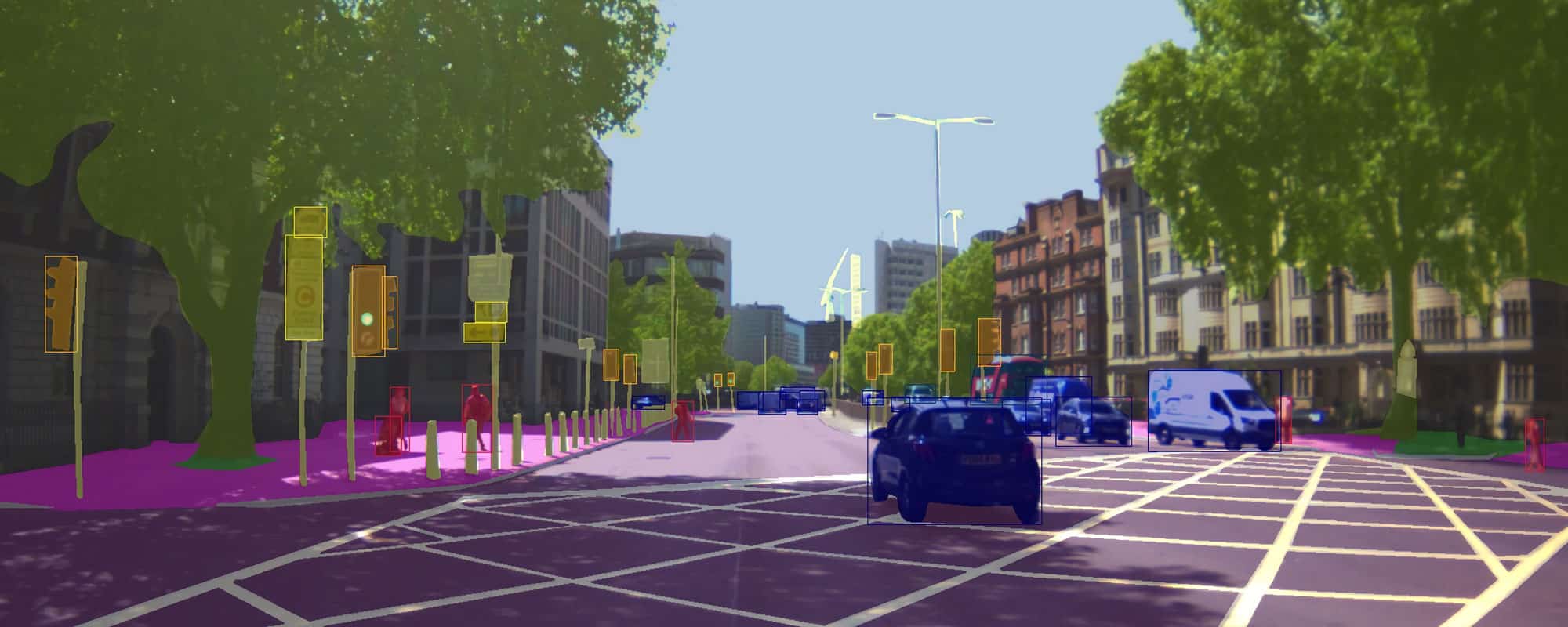
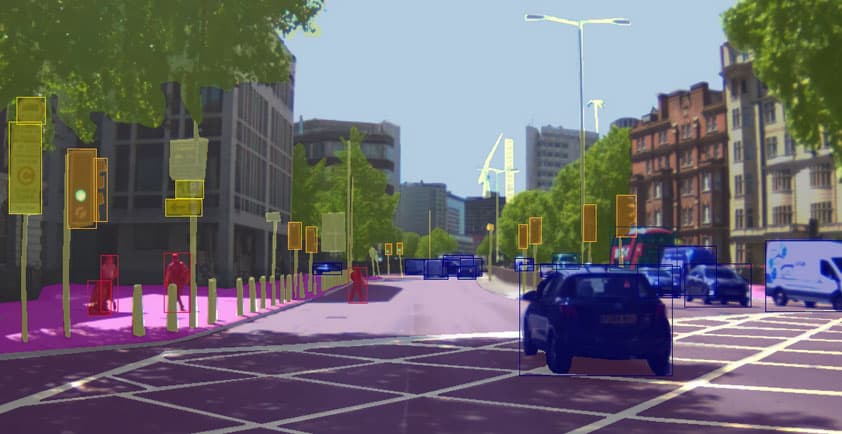
WAYVE CHOOSES MICROSOFT AZURE TO SCALE AI-BASED AUTONOMOUS DRIVING TECHNOLOGY
Wayve, the London-based company that’s developing artificial intelligence software for self-driving cars, has signed a deal with Microsoft to use Azure.
The UK business, which is at the cutting-edge of autonomous development and is seeing strong growth, will use Microsoft’s cloud platform in their research and development of a data-driven solution capable of driving in urban environments.
Wayve started as a member of the Microsoft for Startups: Autonomous Driving program and chose Azure for its reliability, security and ability to cope with large datasets and scale on demand. Azure also includes unique analytics tools that can unlock insight into data and turn it into actionable information to improve businesses.
Alex Kendall, CEO, Wayve said: “Microsoft Azure gives us a secure, agile and reliable cloud platform on which to develop the next generation of urban transport. We are excited by the opportunities that the Azure deal will create for Wayve as we push our machine learning to new levels of scale using data from fleets of connected vehicles across the world.”
Wayve’s computer vision system – the “brain” of the car – learns from observing human driving via reinforcement learning. Traditional self-driving systems rely on expensive Lidar sensors, HD maps and heavy testing in a local area, meaning that they are unable to scale. Wayve’s systems have trained in one city and then successfully driven in a new city they’ve never seen before. This adaptability is why they aim to be the first to deploy self-driving technology in 100 cities.
Michael Wignall, Azure Business Lead at Microsoft, said: “Microsoft is working with Wayve to help it develop advanced technology that could change the way people commute, move around cities and travel long distances. Azure enables a range of companies across many sectors to innovate, collaborate and achieve more, and we are delighted that Wayve has become the latest business to join this group.”


A Wayve vehicle drives down a London street, collecting data via an array of cameras and sensors.
Wayve - Teaming up with Microsoft
With the scale of our ambition to build our Fleet Learning platform to train on diverse and enormous driving data, it became immediately clear we need to move to the cloud.
We built a cost model comparing cloud vs on-premise for our storage and training (GPU-compute) workload, comparing the total cost of ownership over multiple years. We found that with the right architecture, cloud would actually be competitive with on-premise in terms of costs at data scales above 3 petabytes. More importantly, cloud would become more competitive the more data we have, with savings increasing at scale.
So we are going to cloud - but which cloud? It was clear across multiple facets such as cost, technology, and strategic alignment, that Microsoft Azure was the best choice for Wayve.
On cost, Azure was the clear winner, especially due to their option of locally redundant storage (LRS), combined with reserved storage. LRS is a great option for us, as a loss of some of our data would not significantly affect our business, given we are collecting new data continually.
We adopted two tiers of storage for our data: archive and hot. We keep the unfiltered, full-size image data for all cameras in archive storage, whereas hot storage only stores data relevant to our latest training curriculum. For training image (hot) storage, we need very high throughput to keep our GPUs fed. Latency isn't so much a concern, thanks to the pipelined data loading that PyTorch provides us. This allows us to use Azure Blob Storage, greatly simplifying our infrastructure.
We were particularly impressed with Microsoft's innovative approach to deploying the bleeding edge of compute technology into their cloud offering, from Graphcore's IPU to NVIDIA's A100 GPUs. We use a mix of reserved instances and spot (pre-emptible) instances to provide cost effective GPU compute for production training. The reserved instances allow us to meet our base load without fear of eviction, while the spot instances are a great solution for bursty workloads.
Additionally, Azure IoT services provide a lot of infrastructure to support our connected vehicle hardware we deploy across fleets.
Finally, we are inspired to collaborate with Microsoft because of:
> Their existing partnerships within the automotive industry, which we can look to use to commercialise our product,
> Their overall strategy of being a partner and enabler of deep tech, rather than competing directly in the space themselves (e.g. Google/Alphabet & Waymo, Amazon & Zoox),
> Their experience in machine learning at scale through services like Azure Machine
> Learning and partnerships with industry-leading companies.
Putting it all together
In our early beginnings, we showed we can learn an urban driving policy with a small amount of data. We are continuing this research approach to slingshot models into our Fleet Learning Loop: Wayve's driving school, running on Microsoft Azure. This is where we train and evaluate models on petabyte-scale real and synthetic data.
It's our Fleet Learning Loop that will take us from proving an approach is viable with demo-level performance, to human-level, product-worthy autonomy.