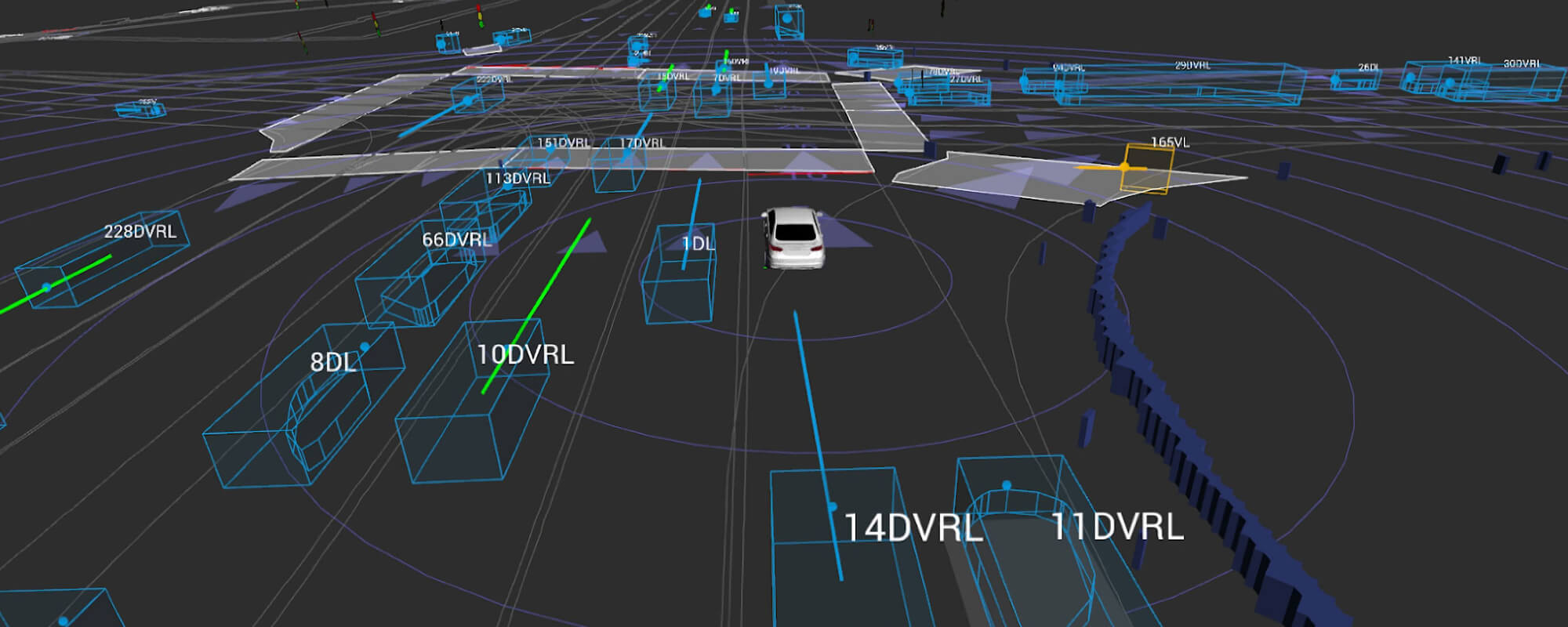
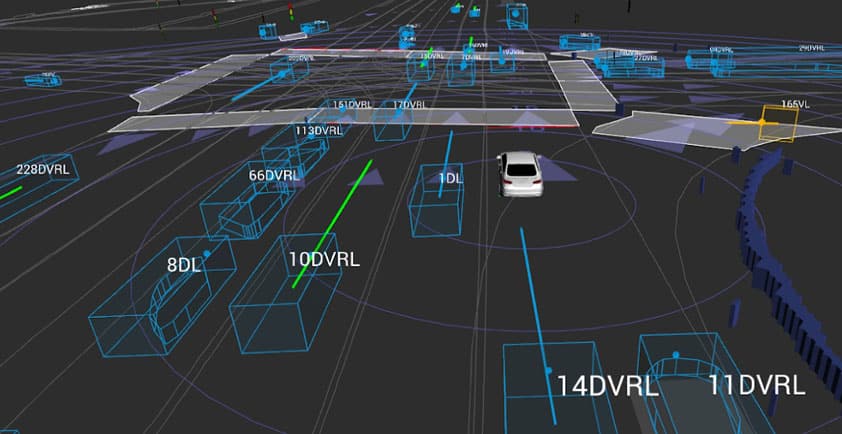
FUELING SELF-DRIVING RESEARCH WITH LEVEL 5’S OPEN PREDICTION DATASET
Given how important and complex self-driving is, we at Lyft deeply care about creating an environment where teams can join forces. To accelerate development and hear from diverse perspectives, we’re collaborating with many stakeholders across the self-driving industry. Today, we are reaching out to the research community. We saw firsthand the community’s engagement with our 2019 perception dataset and competition and are following up with a new challenge.
Today, we’re thrilled to share our self-driving prediction dataset — the largest released to date — and announce our upcoming motion prediction competition.
Data is the fuel for experimenting with the latest machine learning techniques, and limited access to large-scale, quality self-driving data shouldn’t hinder experimentation on this research problem. With this dataset and competition, we aim to empower the research community, accelerate innovation, and share insight on which problems to solve from the perspective of a mature AV program.
Real-world data to solve real-world problems
A key challenge researchers are trying to solve is how to create models that are robust and reliable enough to predict the motion of traffic agents. The Prediction Dataset focuses on this motion prediction problem and includes the movement of many traffic agent types — such as cars, cyclists, and pedestrians — that our AV fleet encountered on our Palo Alto routes. It contains:
> Logs of over 1,000 hours of traffic agent movement
> 170,000 scenes at ~25 seconds long
> 16,000 miles of data collected by 23 self-driving vehicles
> 15,000 semantic map annotations
> The underlying HD semantic map of the area
As our AVs navigate tens of thousands of miles, its sensor suite collects raw camera, lidar, and radar data. Our internal perception stack then processes this raw data stream and generates the logs of traffic movement included in this dataset. When paired with the underlying HD semantic map built by our teams across Palo Alto, London, and Munich, this dataset contains the pieces needed to create the motion prediction models that enable AVs to choose the safest trajectory in a given scenario.
Dataset download and competition
We invite researchers and engineers to download the training dataset and Python-based software kit to begin experimenting with the data—today!
The testing and validation sets will be released as part of our competition, which will kick off in August on the Kaggle platform. Building on the success of last year’s 3D Object Detection competition, we’re encouraging the research community to take on another unsolved AV problem with this year’s competition task: Motion Prediction for AVs. We’ll share more about the prediction problem and the competition during our ECCV workshop on Perception for Autonomous Driving.
We welcome researchers from across the globe to share their solutions and compete for $30,000 in prizes when the competition opens. Subscribe to our competition mailing list to to be notified when it opens. We can’t wait to see what you accomplish.
Building better transportation for everyone, together
We believe self-driving will be a crucial part of a more accessible, safer, and sustainable transportation system. By sharing data with the research community, we hope to illuminate important and unsolved challenges in self-driving. Together, we can make the benefits of self-driving a reality sooner.
See the Predication Dataset Whitepaper.
Author: Sacha Arnoud, Senior Director of Engineering and Peter Ondruska, Head of AV Research